Solving Complex Table Parsing in RAG Systems: A Comparative Analysis
Discover how we compared 8 different parsing solutions to tackle hierarchical tables, merged cells, and horizontally tiled tables in PDFs for RAG applications.
RAG over PDFs containing complex tables has consistently posed a significant challenge. Developers experiment with various parsing solutions, but identifying the most effective parser for specific use cases remains tedious. Recently, we tackled a particularly challenging parsing scenario for one of our client's RAG use cases.
Key Parsing Challenges:
- Hierarchical Tables: Tables with nested headings and multiple levels of subheadings.
- Merged Cells: Merged cells spanning multiple rows or columns. Determining how to accurately assign merged cell values to all relevant cells was crucial.
- Horizontally Tiled Tables: Differentiating between separate tables placed side by side was essential to prevent treating horizontally aligned tables as a single, contiguous table.
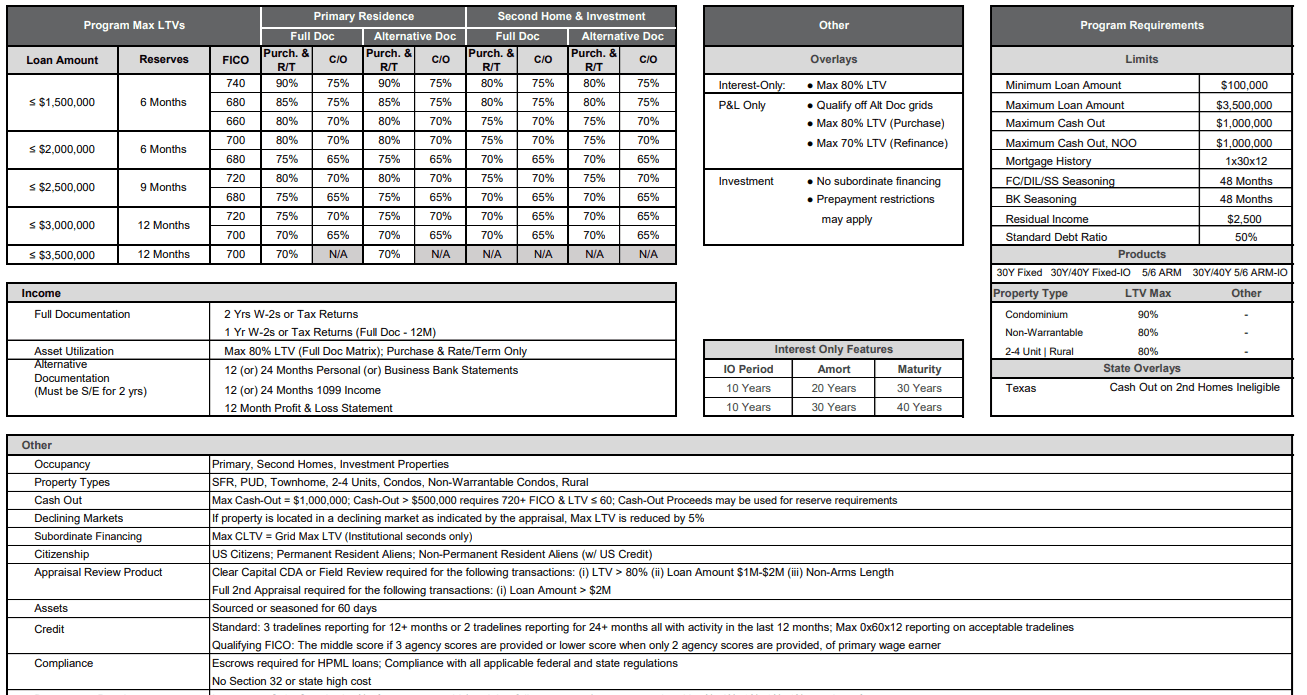
- Basic Python parser
- GPT-4o
- Gemini 2.0 Flash
- Claude 3.5
- Claude 3.7
- Unstructured.io
- LlamaParse Premium mode
- LlamaParse Layout Agent
Test Questions:
- Question 1: What is the minimum FICO score required for a $1.8 million loan?
- Expected Answer: 680
- Question 2: For a second home purchase transaction using full documentation, with a loan amount of $2.4 million and borrower FICO score of 730, what is the maximum allowable LTV?
- Expected Answer: 75%
- Question 3: What is the maximum allowable LTV for a $3.1 million cash-out loan on a primary residence using alternative documentation?
- Expected Answer: Cash-out loans under these circumstances are not permitted.
Results:
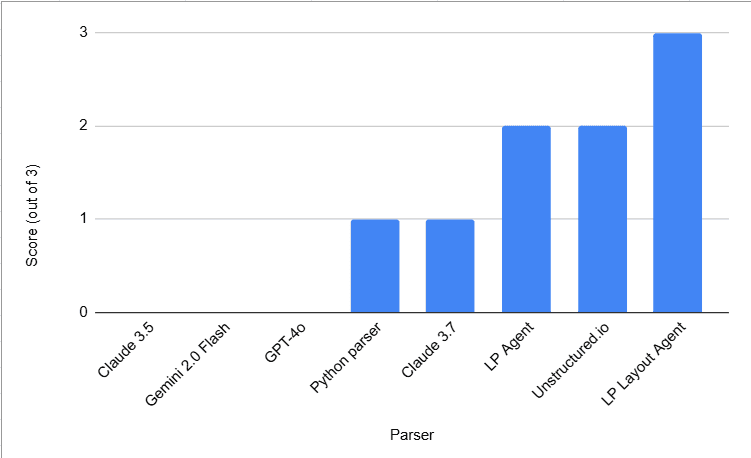
The detailed responses of Claude for each of the above parsers are given here.
Related Posts
Why Building Production-Grade RAG Applications Is So Hard
Learn why creating demo RAG applications is easy, but building production-grade systems is exponentially harder, and how Queryloop solves these challenges.
Automating RAG Optimization: Finding Optimal Configurations Through Systematic Testing
Learn how Queryloop automates RAG optimization through systematic testing of parameter combinations to maximize accuracy, minimize latency, and control costs for complex document analysis.